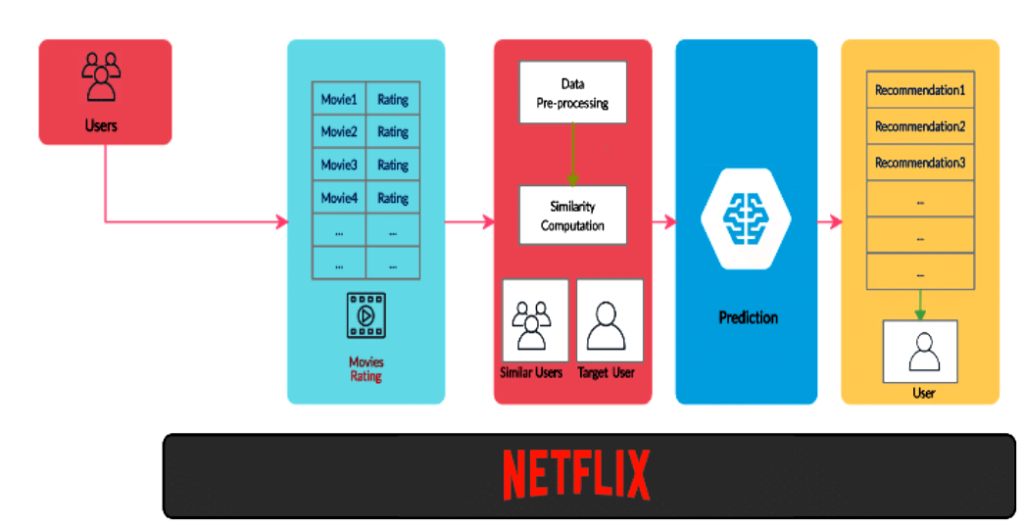
Introduction
When it comes to understanding the unparalleled might of data-driven personalization, one cannot bypass the behemoth of bespoke content delivery: Netflix. With a subscriber base sprawling across 190 countries and an exhaustive library of content, the streaming giant continually excels at keeping its diverse audience engaged. The linchpin of this enviable engagement? A sophisticated recommendation engine that materializes the pinnacle of personalization.
Unlocking Viewer Preferences
Netflix allocates a whopping $1 billion annually to its recommendation system, underscoring a commitment to understanding and predicting viewer preferences. This investment propels machine learning algorithms that delve into a complex web of user interactions, viewing histories, and content attributes. But the magic lies in interpreting this data in a way that, almost intuitively, knows a user’s next binge-worthy series or under-the-radar film gem.
Collaborative Filtering a Confluence of Likes and Dislikes
Collaborative filtering forms the bedrock of Netflix’s recommendation prowess, navigating through vast data oceans to link similar user behaviors and preferences. The model operates on two pivotal frameworks: User-User and Item-Item collaborative filtering. While the former pairs users with analogous viewing habits, the latter aligns items that have been similarly rated or interacted with, ensuring that the content nudged towards a viewer is reflective of their intrinsic and evolving tastes.
Dive into Deep Learning
Further refinement of content suggestions is manifested through deep learning techniques that scrutinize viewing patterns with astonishing precision. By implementing neural networks, Netflix deciphers subtle patterns and nuanced preferences that might elude traditional predictive models, orchestrating a content symphony that aligns with both overt and covert user desires.
Contextual Bandit Balancing Exploration and Exploitation
Netflix negotiates a delicate balancing act between offering tried-and-true favorites and introducing viewers to novel content through a method known as the contextual bandit algorithm. This mechanism strives to optimize a fine balance between exploiting known viewer preferences and exploring uncharted content territories, ensuring that the user experience is a harmonious blend of comfort and curiosity.
The Art and Science of Thumbnails
The recommendation engine’s wizardry extends beyond mere content suggestions to encompass aesthetic appeals through dynamic thumbnails. By analyzing which images, a user is more inclined to click on, Netflix optimizes thumbnail presentations to augment appeal and, by extension, engagement.
Results A Symphony of Science and Satisfaction
The tangible impact of Netflix’s recommendation engine isn’t merely speculative. A staggering 80% of hours streamed are from automated recommendations, a testament to the engine’s efficacy in curating content that resonates and retains. Moreover, personalized content curations have substantially mitigated churn, safeguarding Netflix’s position as a streaming titan amidst a sea of burgeoning competitors.
Conclusion
Netflix has harmoniously blended technology and viewer psychology to orchestrate a recommendation engine that not only understands but anticipates user preferences. This intricate dance of data, algorithms, and user experience forms a lustrous tapestry that enchants viewers and secures unwavering loyalty in a fluctuating digital landscape. For marketers and technologists alike, Netflix’s saga of personalization underscores the boundless potentials of marrying data, technology, and an unerring commitment to user satisfaction.
This succinct exploration into Netflix’s recommendation engine underscores the expansive and intricate technological frameworks that underpin its globally renowned personalization capabilities, offering insights and inspiration for marketing and technology professionals navigating the complex terrains of user engagement and content delivery in the digital age.